The AI Balancing Act in Financial GRC: Navigating Compliance, Risk, and Innovation
- NTM Team
- Apr 28
- 3 min read
Financial institutions face a complex challenge in adopting AI for governance, risk, and compliance (GRC): balancing efficiency gains with regulatory scrutiny and operational risks. While a large number of financial firms are exploring AI for GRC, only 32% have established an AI committee or governance group, exposing critical gaps in oversight. Below, we dissect the key challenges and strategies for integrating AI into financial GRC responsibly.
Core Challenges in AI-Driven GRC
1. Governance Deficits
Third-party risks: 92% of firms lack policies for managing AI tools from external vendors, creating vulnerabilities in supply chains and compliance.
Fragmented oversight: Few organizations have cross-functional AI councils to align IT, legal, and compliance teams, leading to siloed risk management.
2. Model Validation Complexities
Black box dilemma: Many AI/ML models lack transparency, complicating validation for anti-money laundering (AML) and fraud detection systems.
Regulatory demands: The OCC mandates three validation pillars—conceptual soundness, ongoing monitoring, and outcomes analysis3—which are harder to implement with AI’s dynamic decision-making.
3. Regulatory Fragmentation
Global divergence: Institutions must comply with 15+ overlapping frameworks, including the EU AI Act, U.S. SEC guidelines, and Japan’s draft AI rules.
Real-time compliance: Regulators increasingly demand continuous monitoring instead of periodic audits, straining legacy systems.
4. Data and Talent Shortages
Quality gaps: 87% of GRC teams report siloed or incomplete data, leading to 42% more false positives in compliance alerts.
Skill deficits: 68% of firms lack staff with combined AI and regulatory expertise, slowing implementation.
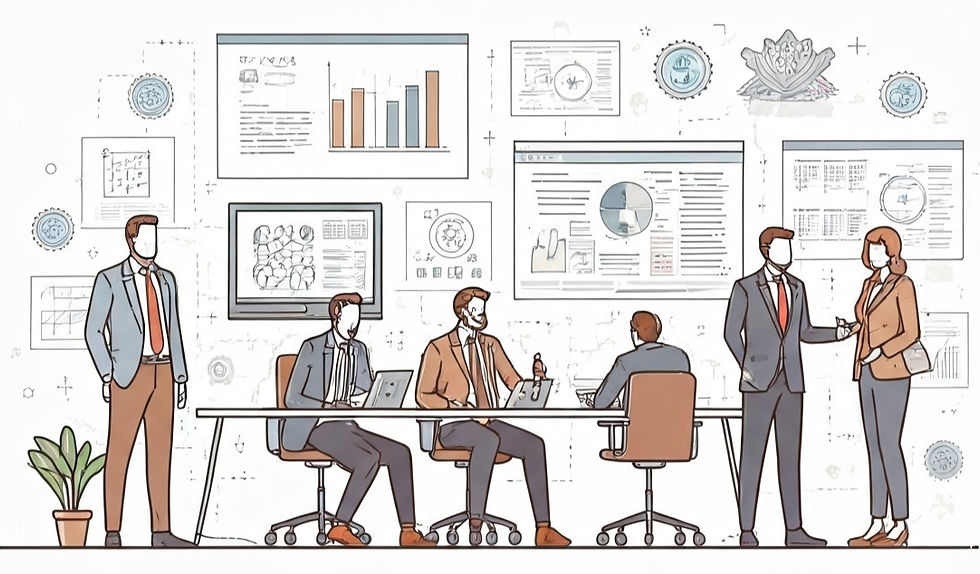
Strategic Solutions
A. Enhanced Governance Frameworks
AI risk committees: Integrate cross-departmental teams to oversee model development, third-party tools, and ethical AI use.
Vendor AI audits: Mandate contractual obligations for explainability and bias mitigation in third-party AI systems.
B. Explainable AI (XAI) for Validation
Traditional Validation | AI-Driven Validation |
Annual manual reviews | Continuous automated monitoring |
Rule-based outputs | Natural language decision narrative |
Static risk assessments | Real-time anomaly detection |
C. Regulatory Adaptation Tools
AI compliance mapping: Deploy NLP to auto-align controls with 2,300+ annual regulatory updates.
Regulatory sandboxes: Test AI tools in controlled environments with regulatory approval to preempt compliance issues.
JPMorgan Chase reduced false fraud positives by 50% and achieved a 95% reduction in AML false positives using AI that maps transaction patterns across 120,000 regulatory sources.
D. Data Standardization and Upskilling
Centralize GRC data lakes with unified schemas
Implement bias detection algorithms for high-risk models
Train “AI translators” to bridge technical and compliance teams
The Path Forward
Financial institutions that successfully balance AI innovation with compliance management report 35% faster audit cycles and 50% lower remediation costs. Critical steps include:
Allocating 15–20% of GRC budgets to AI-specific risk management
Adopting ISO 42001-like standards for AI governance
Implementing phased rollouts starting with high-impact areas like fraud detection
As the EU’s Digital Operational Resilience Act (DORA) and U.S. AI Executive Orders take effect in 2026, proactive firms will leverage AI not just for efficiency but as a strategic differentiator in regulatory trust.
Note: This analysis synthesizes regulatory texts, industry surveys, and technical guides to provide a roadmap for financial GRC teams navigating AI adoption. This report is for educational purposes only and is not intended to be legal advice. By prioritizing explainability, governance, and adaptive compliance, institutions can turn AI’s risks into competitive advantages.
コメント